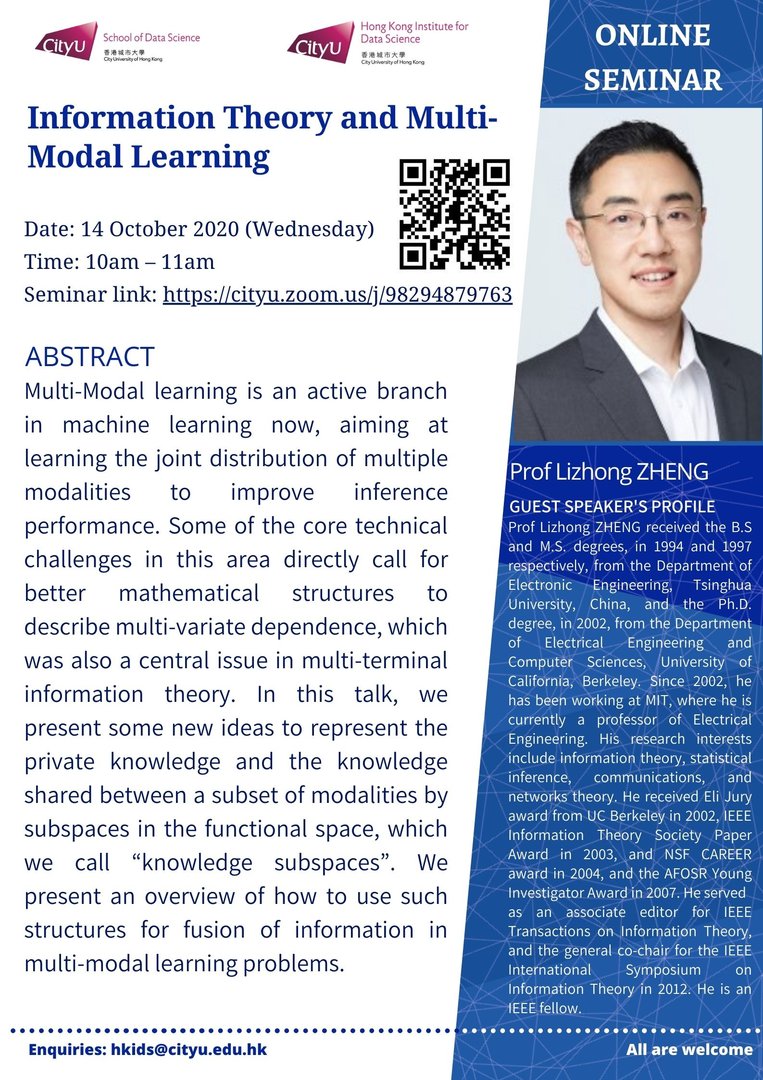
Multi-Modal learning is an active branch in machine learning now, aiming at learning the joint distribution of multiple modalities to improve inference performance. Some of the core technical challenges in this area directly call for better mathematical structures to describe multi-variate dependence, which was also a central issue in multi-terminal information theory. In this talk, we present some new ideas to represent the private knowledge and the knowledge shared between a subset of modalities by subspaces in the functional space, which we call “knowledge subspaces”. We present an overview of how to use such structures for fusion of information in multi-modal learning problems.
Speaker: Professor Lizhong ZHENG
Date: 14 October 2020 (Wed)
Time: 10am - 11am
Poster: Click here
Latest Seminar
Biography
Prof Lizhong ZHENG received the B.S and M.S. degrees, in 1994 and 1997 respectively, from the Department of Electronic Engineering, Tsinghua University, China, and the Ph.D. degree, in 2002, from the Department of Electrical Engineering and Computer Sciences, University of California, Berkeley. Since 2002, he has been working at MIT, where he is currently a professor of Electrical Engineering. His research interests include information theory, statistical inference, communications, and networks theory. He received Eli Jury award from UC Berkeley in 2002, IEEE Information Theory Society Paper Award in 2003, and NSF CAREER award in 2004, and the AFOSR Young Investigator Award in 2007. He served as an associate editor for IEEE Transactions on Information Theory, and the general co-chair for the IEEE International Symposium on Information Theory in 2012. He is an IEEE fellow.