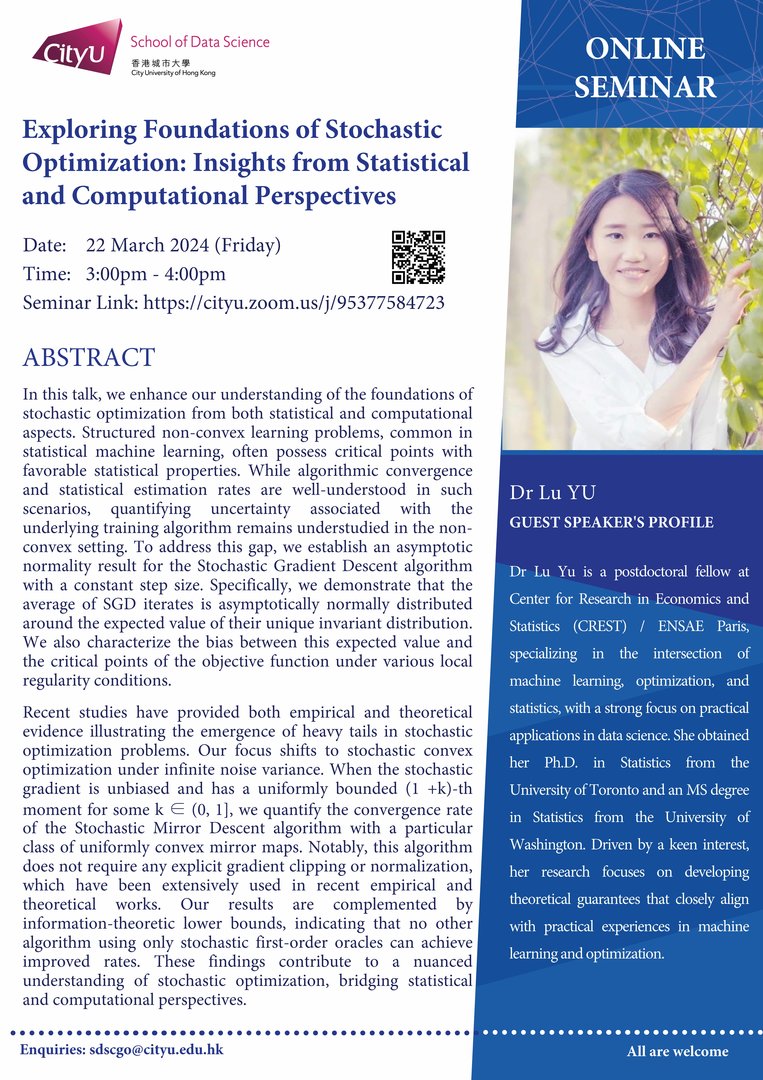
n this talk, we enhance our understanding of the foundations of stochastic optimization from both statistical and computational aspects. Structured non-convex learning problems, common in statistical machine learning, often possess critical points with favorable statistical properties. While algorithmic convergence and statistical estimation rates are well-understood in such scenarios, quantifying uncertainty associated with the underlying training algorithm remains understudied in the non-convex setting. To address this gap, we establish an asymptotic normality result for the Stochastic Gradient Descent algorithm with a constant step size. Specifically, we demonstrate that the average of SGD iterates is asymptotically normally distributed around the expected value of their unique invariant distribution. We also characterize the bias between this expected value and the critical points of the objective function under various local regularity conditions.
Recent studies have provided both empirical and theoretical evidence illustrating the emergence of heavy tails in stochastic optimization problems. Our focus shifts to stochastic convex optimization under infinite noise variance. When the stochastic gradient is unbiased and has a uniformly bounded (1 +k)-th moment for some k ∈ (0, 1], we quantify the convergence rate of the Stochastic Mirror Descent algorithm with a particular class of uniformly convex mirror maps. Notably, this algorithm does not require any explicit gradient clipping or normalization, which have been extensively used in recent empirical and theoretical works. Our results are complemented by information-theoretic lower bounds, indicating that no other algorithm using only stochastic first-order oracles can achieve improved rates. These findings contribute to a nuanced understanding of stochastic optimization, bridging statistical and computational perspectives.
Speaker: Dr Lu YU
Date: 22 March 2024 (Friday)
Time: 3:00pm – 4:00pm
Poster: Click here
Latest Seminar
Biography
Dr Lu Yu is a postdoctoral fellow at Center for Research in Economics and Statistics (CREST) / ENSAE Paris, specializing in the intersection of machine learning, optimization, and statistics, with a strong focus on practical applications in data science. She obtained her Ph.D. in Statistics from the University of Toronto and an MS degree in Statistics from the University of Washington. Driven by a keen interest, her research focuses on developing theoretical guarantees that closely align with practical experiences in machine learning and optimization.