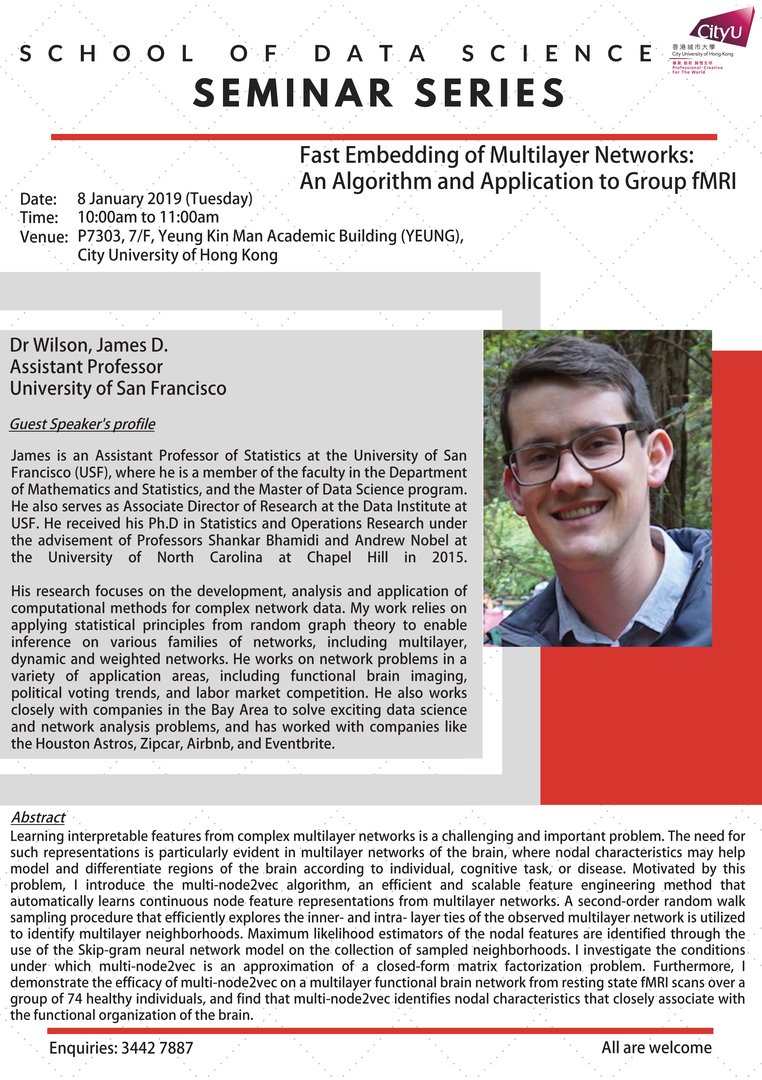
Learning interpretable features from complex multilayer networks is a challenging and important problem. The need for such representations is particularly evident in multilayer networks of the brain, where nodal characteristics may help model and differentiate regions of the brain according to individual, cognitive task, or disease. Motivated by this problem, I introduce the multi-node2vec algorithm, an efficient and scalable feature engineering method that automatically learns continuous node feature representations from multilayer networks. A second-order random walk sampling procedure that efficiently explores the inner- and intra- layer ties of the observed multilayer network is utilized to identify multilayer neighborhoods. Maximum likelihood estimators of the nodal features are identified through the use of the Skip-gram neural network model on the collection of sampled neighborhoods. I investigate the conditions under which multi-node2vec is an approximation of a closed-form matrix factorization problem. Furthermore, I demonstrate the efficacy of multi-node2vec on a multilayer functional brain network from resting state fMRI scans over a group of 74 healthy individuals, and find that multi-node2vec identifies nodal characteristics that closely associate with the functional organization of the brain.
Date: 8 January 2019 (Tue)
Time: 10:00am - 11:00am
Speaker: Dr James D. Wilson
Poster: Click here
Latest Seminar
Biography
James is an Assistant Professor of Statistics at the University of San Francisco (USF), where he is a member of the faculty in the Department of Mathematics and Statistics, and the Master of Data Science program. He also serves as Associate Director of Research at the Data Institute at USF. He received his Ph.D in Statistics and Operations Research under the advisement of Professors Shankar Bhamidi and Andrew Nobel at the University of North Carolina at Chapel Hill in 2015. His research focuses on the development, analysis and application of computational methods for complex network data. My work relies on applying statistical principles from random graph theory to enable inference on various families of networks, including multilayer, dynamic and weighted networks. He works on network problems in a variety of application areas, including functional brain imaging, political voting trends, and labor market competition. He also works closely with companies in the Bay Area to solve exciting data science and network analysis problems, and has worked with companies like the Houston Astros, Zipcar, Airbnb, and Eventbrite.