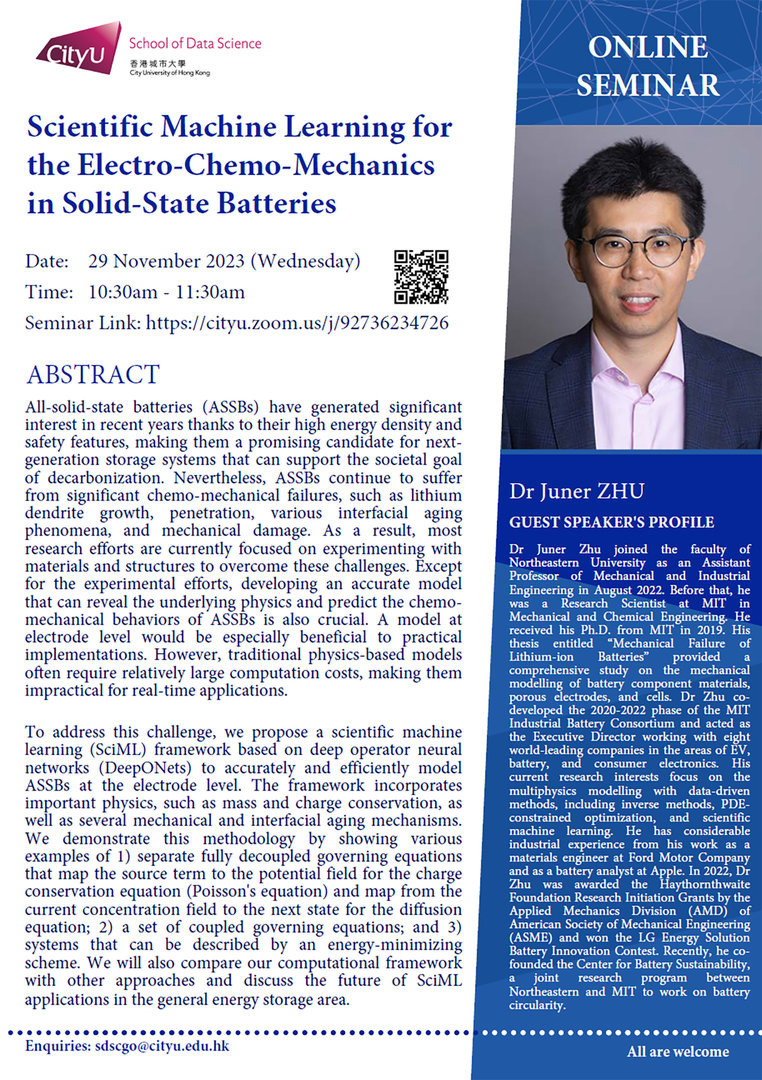
All-solid-state batteries (ASSBs) have generated significant interest in recent years thanks to their high energy density and safety features, making them a promising candidate for next-generation storage systems that can support the societal goal of decarbonization. Nevertheless, ASSBs continue to suffer from significant chemo-mechanical failures, such as lithium dendrite growth, penetration, various interfacial aging phenomena, and mechanical damage. As a result, most research efforts are currently focused on experimenting with materials and structures to overcome these challenges. Except for the experimental efforts, developing an accurate model that can reveal the underlying physics and predict the chemo-mechanical behaviors of ASSBs is also crucial. A model at electrode level would be especially beneficial to practical implementations. However, traditional physics-based models often require relatively large computation costs, making them impractical for real-time applications.
To address this challenge, we propose a scientific machine learning (SciML) framework based on deep operator neural networks (DeepONets) to accurately and efficiently model ASSBs at the electrode level. The framework incorporates important physics, such as mass and charge conservation, as well as several mechanical and interfacial aging mechanisms. We demonstrate this methodology by showing various examples of 1) separate fully decoupled governing equations that map the source term to the potential field for the charge conservation equation (Poisson's equation) and map from the current concentration field to the next state for the diffusion equation; 2) a set of coupled governing equations; and 3) systems that can be described by an energy-minimizing scheme. We will also compare our computational framework with other approaches and discuss the future of SciML applications in the general energy storage area.
Speaker: Dr Juner ZHU
Date: 29 November 2023 (Wednesday)
Time: 10:30am – 11:30am
Poster: Click here
Latest Seminar
Biography
Dr Juner Zhu joined the faculty of Northeastern University as an Assistant Professor of Mechanical and Industrial Engineering in August 2022. Before that, he was a Research Scientist at MIT in Mechanical and Chemical Engineering. He received his Ph.D. from MIT in 2019. His thesis entitled “Mechanical Failure of Lithium-ion Batteries” provided a comprehensive study on the mechanical modelling of battery component materials, porous electrodes, and cells. Dr Zhu co-developed the 2020-2022 phase of the MIT Industrial Battery Consortium and acted as the Executive Director working with eight world-leading companies in the areas of EV, battery, and consumer electronics. His current research interests focus on the multiphysics modelling with data-driven methods, including inverse methods, PDE-constrained optimization, and scientific machine learning. He has considerable industrial experience from his work as a materials engineer at Ford Motor Company and as a battery analyst at Apple. In 2022, Dr Zhu was awarded the Haythornthwaite Foundation Research Initiation Grants by the Applied Mechanics Division (AMD) of American Society of Mechanical Engineering (ASME) and won the LG Energy Solution Battery Innovation Contest. Recently, he co-founded the Center for Battery Sustainability, a joint research program between Northeastern and MIT to work on battery circularity.