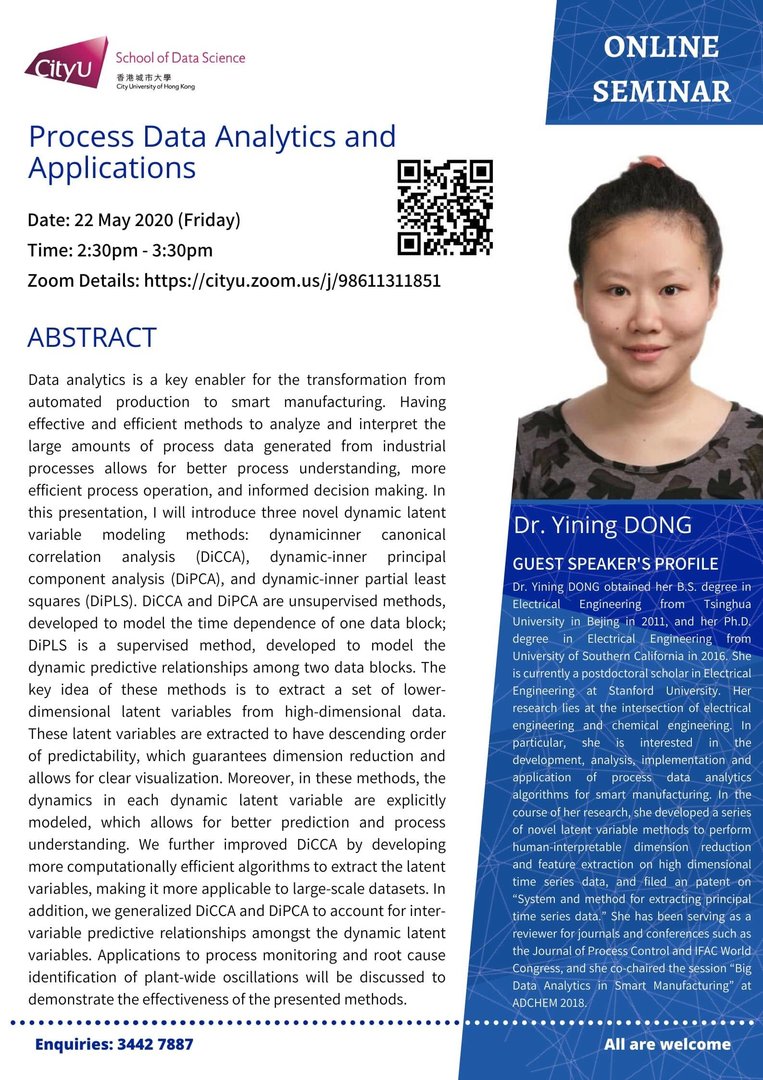
Data analytics is a key enabler for the transformation from automated production to smart manufacturing. Having effective and efficient methods to analyze and interpret the large amounts of process data generated from industrial processes allows for better process understanding, more efficient process operation, and informed decision making. In this presentation, I will introduce three novel dynamic latent variable modeling methods: dynamicinner canonical correlation analysis (DiCCA), dynamic-inner principal component ana lysis (DiPCA), and dynamic-inner partial least squares (DiPLS). DiCCA and DiPCA are unsupervised methods, developed to model the time dependence of one data block; DiPLS is a supervised method, developed to model the dynamic predictive relationships among two data blocks. The key idea of these methods is to extract a set of lower dimensional latent variab les from high-dimensional data. These latent var iables are extracted to have descending order of predictability, which guarantees dimension reduction and allows for clear visualization. Moreover, in these methods,the dynamics in each dynamic latent variable are explicitly modeled, which allows for better prediction and process understanding. We further improved DiCCA by developing more computat ionally efficient algorithms to extract the latent variables, making it more applicable to large-scale datasets. In addition, we genera lized DiCCA and DiPCA to account for inter variable predictive relationships amongst the dynamic latent variables. Applications to process monitoring and root cause identification of plant-wide oscillations will be discussed to demonstrate the effectiveness of the presented methods.
Speaker: Dr Yining DONG
Date: 22 May 2020 (Fri)
Time: 14:30pm - 15:30pm
Poster: Click here
Latest Seminar
Biography
Dr. Yining DONG obtained her B.S. degree in Electrical Engineering from Tsinghua University in Beijing in 2011, and her Ph.D. degree in Electrical Engineering from University of Southern California in 2016. She is currently a postdoctoral scholar in Electrical Engineering at Standford University. Her research lies at the intersaction of electrical engineering and chemical engineering. In particular, she is interested in the development, analysis, implementation and application of process data analytics algorithms for smart manufacturing. In the course of her research, she developed a series of novel latent variable methods to perform human-interpretable dimension reduction and feature extraction on high dimensional time series data, and filed on patent on "System and method for extracting principal time series data". She has been serving as a reviewer for journals and conferences such as the Journal of Process Control and IFAC World Congress, and she co-chaired the session "Big Data Analytics in Smart Manufacturing" at ADCHEM 2018.