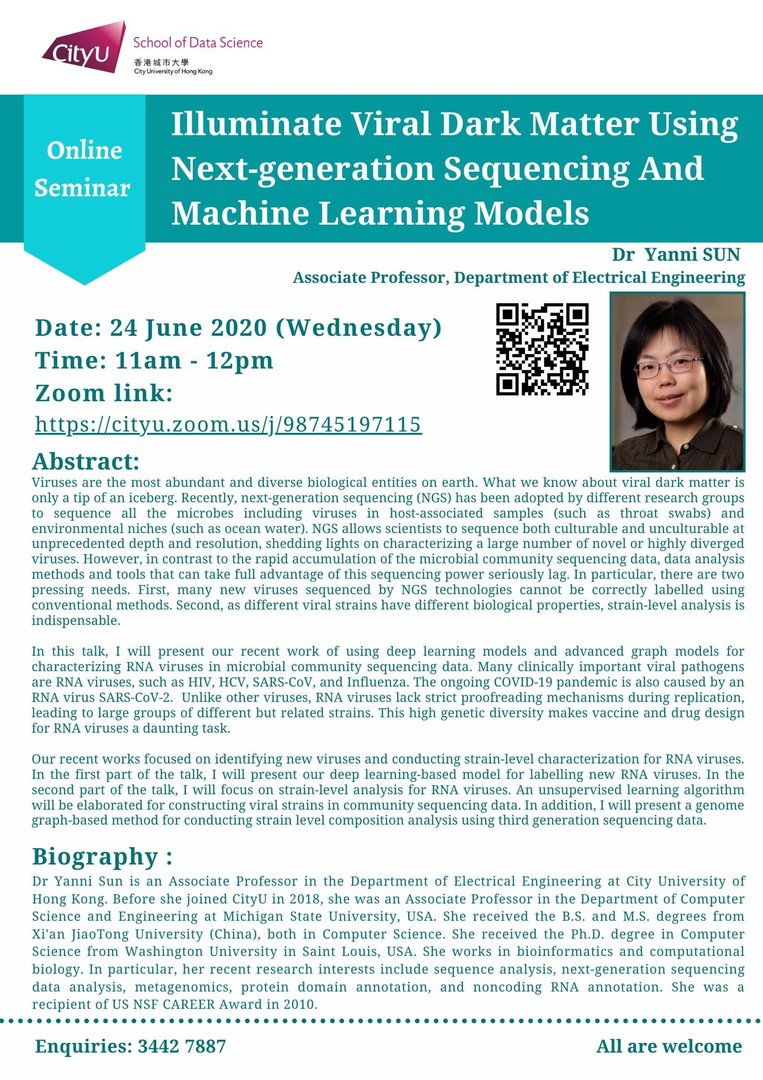
Viruses are the most abundant and diverse biological entities on earth. What we know about viral dark matter is only a tip of an iceberg. Recently, next-generation sequencing (NGS) has been adopted by different research groups to sequence all the microbes including viruses in host-associated samples (such as throat swabs) and environmental niches (such as ocean water). NGS allows scientists to sequence both culturable and unculturable at unprecedented depth and resolution, shedding lights on characterizing a large number of novel or highly diverged viruses. However, in contrast to the rapid accumulation of the microbial community sequencing data, data analysis methods and tools that can take full advantage of this sequencing power seriously lag. In particular, there are two pressing needs. First, many new viruses sequenced by NGS technologies cannot be correctly labelled using conventional methods. Second, as different viral strains have different biological properties, strain-level analysis is indispensable .
In this talk, I will present our recent work of using deep learning models and advanced graph models for characterizing RNA viruses in microbial community sequencing data. Many clinically important viral pathogens are RNA viruses, such as HIV, HCV, SARS-CoV, and Influenza. The ongoing COVID-19 pandemic is also caused by an RNA virus SARS-CoV-2. Unlike other viruses, RNA viruses lack strict proofreading mechanisms during replication , leading to large groups of different but related strains. This high genetic diversity makes vaccine and drug design for RNA viruses a daunting task.
Our recent works focused on identifying new viruses and conducting strain-level characterization for RNA viruses. In the first part of the talk, I will present our deep learning-based model for labelling new RNA viruses. In the second part of the talk, I will focus on strain-level analysis for RNA viruses. An unsupervised learning algorithm will be elaborated for constructing viral strains in community sequencing data. In addition, I will present a genome graph-based method for conducting strain level composition analysis using third generation sequencing data.
Speaker: Dr Yanni SUN
Date: 24 June 2020 (Wed)
Time: 11:00am - 12:00pm
Poster: Click here
Latest Seminar
Biography
Dr Yanni Sun is an Associate Professor in the Depa rtment of Electrical Engineering at City University of Hong Kong. Before she joined CityU in 2018, she was an Associate Professor in the Department of Computer Science and Engineering at Michigan State University, USA. She received the B.S. and M.S. degrees from Xi'an JiaoTong University (China), both in Computer Science. She received the Ph.D. degree in Computer Science from Washington University in Saint Louis, USA. She works in bioinformatics and computational biology. In particular, her recent research interests include sequence analysis, next-generation sequencing data analysis, metagenomics, protein domain annotation, and noncoding RNA annotation. She was a recipient of US NSF CAREER Award in 2010.