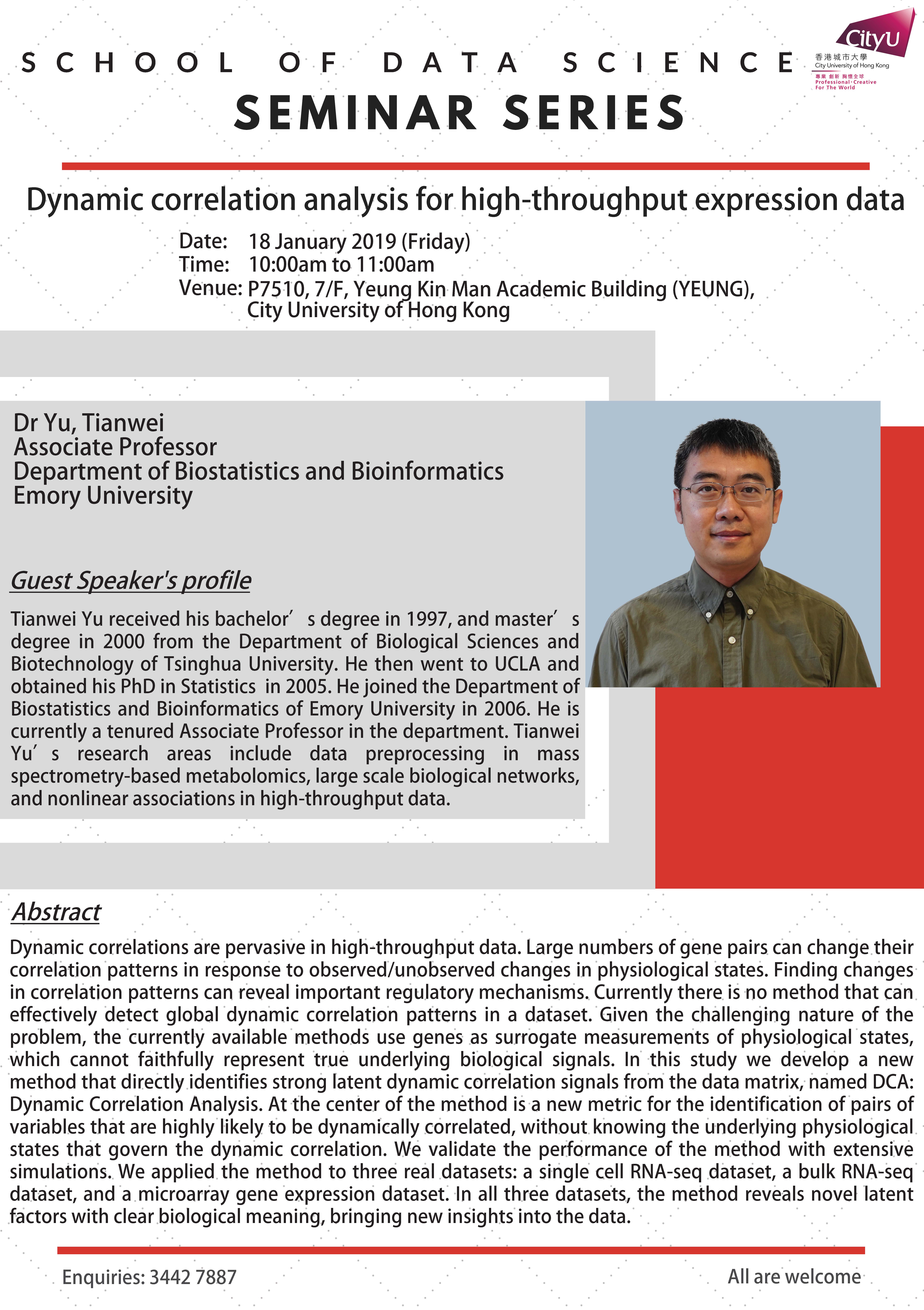
Dynamic correlations are pervasive in high-throughput data. Large numbers of gene pairs can change their correlation patterns in response to observed/unobserved changes in physiological states. Finding changes in correlation patterns can reveal important regulatory mechanisms. Currently there is no method that can effectively detect global dynamic correlation patterns in a dataset. Given the challenging nature of the problem, the currently available methods use genes as surrogate measurements of physiological states, which cannot faithfully represent true underlying biological signals. In this study we develop a new method that directly identifies strong latent dynamic correlation signals from the data matrix, named DCA: Dynamic Correlation Analysis. At the center of the method is a new metric for the identification of pairs of variables that are highly likely to be dynamically correlated, without knowing the underlying physiological states that govern the dynamic correlation. We validate the performance of the method with extensive simulations. We applied the method to three real datasets: a single cell RNA-seq dataset, a bulk RNA-seq dataset, and a microarray gene expression dataset. In all three datasets, the method reveals novel latent factors with clear biological meaning, bringing new insights into the data.
Date: 18 January 2019 (Fri)
Time: 10:00am - 11:00am
Speaker: Dr Tianwei YU
Poster: Click here
Latest Seminar
Biography
Dr Tianwei Yu received his bachelor’s degree in 1997, and master’s degree in 2000 from the Department of Biological Sciences and Biotechnology of Tsinghua University. He then went to UCLA and obtained his PhD in Statistics in 2005. He joined the Department of Biostatistics and Bioinformatics of Emory University in 2006. He is currently a tenured Associate Professor in the department. Tianwei Yu’s research areas include data preprocessing in mass spectrometry-based metabolomics, large scale biological networks, and nonlinear associations in high-throughput data.