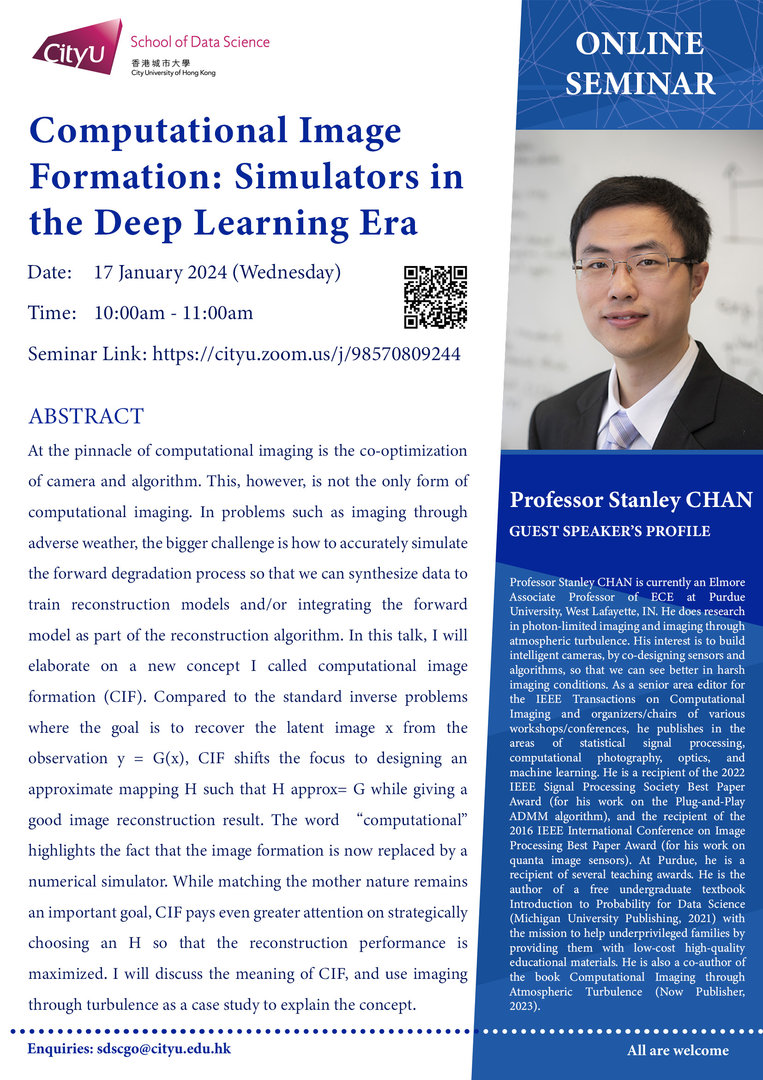
At the pinnacle of computational imaging is the co-optimization of camera and algorithm. This, however, is not the only form of computational imaging. In problems such as imaging through adverse weather, the bigger challenge is how to accurately simulate the forward degradation process so that we can synthesize data to train reconstruction models and/ or integrating the forward model as part of the reconstruction algorithm. In this talk, I will elaborate on a new concept I called computational image formation (CIF). Compared to the standard inverse problems where the goal is to recover the latent image x from the observation y = G(x), CIF shifts the focus to designing an approximate mapping H such that H approx= G while giving a good image reconstruction result. The word "computational" highlights the fact that the image formation is now replaced by a numerical simulator. While matching the mother nature remains an important goal, CIF pays even greater attention on strategically choosing an H so that the reconstruction performance is maximized. I will discuss the meaning of CIF, and use imaging through turbulence as a case study to explain the conce抖
Speaker: Professor Stanley Chan
Date: 17 January 2024 (Wednesday)
Time: 10:00am – 11:00am
Poster: Click here
Latest Seminar
Biography
Professor Stanley CHAN is currently an Elmore Associate Professor of ECE at Purdue University, West Lafayette, IN. He does research in photon-limited imaging and imaging through atmospheric turbulence. His interest is to build intelligent cameras, by co-designing sensors and algorithms, so that we can see better in harsh imaging conditions. As a senior area editor for the IEEE Transactions on Computational Imaging and organizers/chairs of various workshops/conferences, he publishes in the areas of statistical signal processing, computational photography, optics, and machine learning. He is a recipient of the 2022 IEEE Signal Processing Society Best Paper Award (for his work on the Plug-and-Play ADMM algorithm), and the recipient of the 2016 IEEE International Conference on Image Processing Best Paper Award (for his work on quanta image sensors). At Purdue, he is a recipient of several teaching awards. He is the author of a free undergraduate textbook Introduction to Probability for Data Science (Michigan University Publishing, 2021) with the mission to help underprivileged families by providing them with low-cost high-quality educational materials. He is also a co-author of the book Computational Imaging through Atmospheric Turbulence (Now Publisher, 2023).