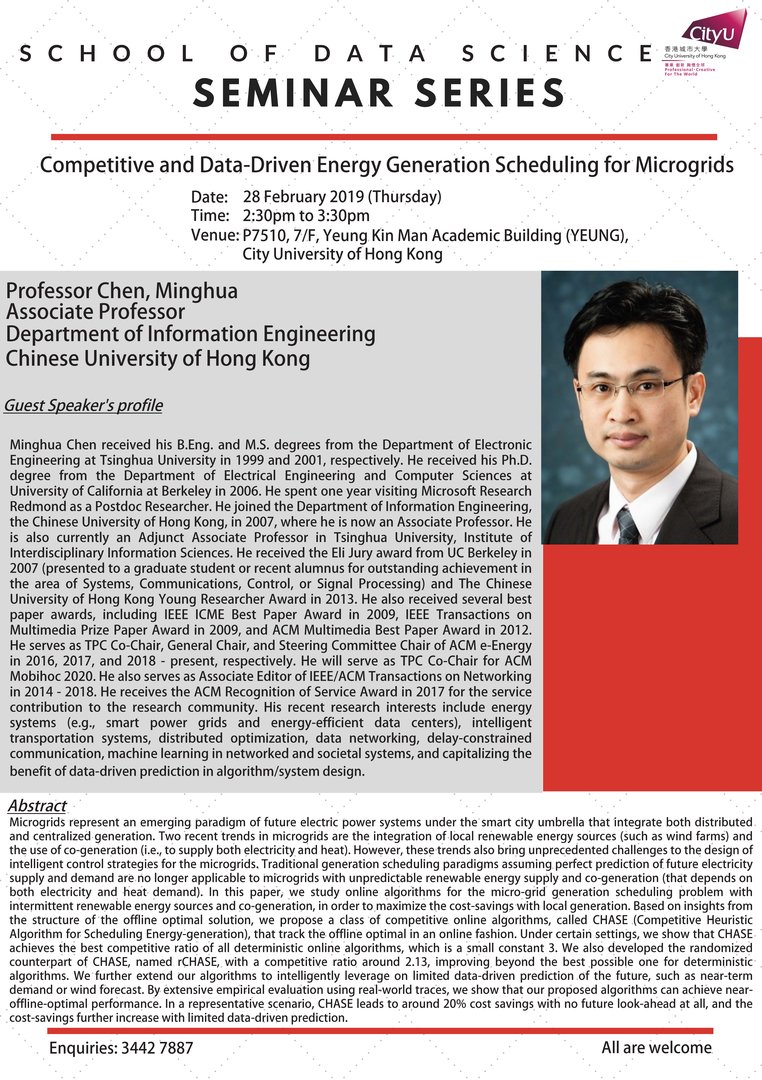
Microgrids represent an emerging paradigm of future electric power systems under the smart city umbrella that integrate both distributed and centralized generation. Two recent trends in microgrids are the integration of local renewable energy sources (such as wind farms) and the use of co-generation (i.e., to supply both electricity and heat). However, these trends also bring unprecedented challenges to the design of intelligent control strategies for the microgrids. Traditional generation scheduling paradigms assuming perfect prediction of future electricity supply and demand are no longer applicable to microgrids with unpredictable renewable energy supply and co-generation (that depends on both electricity and heat demand). In this paper, we study online algorithms for the micro-grid generation scheduling problem with intermittent renewable energy sources and co-generation, in order to maximize the cost-savings with local generation. Based on insights from the structure of the offline optimal solution, we propose a class of competitive online algorithms, called CHASE (Competitive Heuristic Algorithm for Scheduling Energy-generation), that track the offline optimal in an online fashion. Under certain settings, we show that CHASE achieves the best competitive ratio of all deterministic online algorithms, which is a small constant 3. We also developed the randomized counterpart of CHASE, named rCHASE, with a competitive ratio around 2.13, improving beyond the best possible one for deterministic algorithms. We further extend our algorithms to intelligently leverage on limited data-driven prediction of the future, such as near-term demand or wind forecast. By extensive empirical evaluation using real-world traces, we show that our proposed algorithms can achieve near-offline-optimal performance. In a representative scenario, CHASE leads to around 20% cost savings with no future look-ahead at all, and the cost-savings further increase with limited data-driven prediction.
Speaker: Professor Chen Minghua, Associate Professor
Date: 28 February 2019
Time: 14:30pm - 15:30pm
Poster: Click here
Latest Seminar
Biography
Prof Minghua Chen received his B.Eng. and M.S. degrees from the Department of Electronic Engineering at Tsinghua University in 1999 and 2001, respectively. He received his Ph.D. degree from the Department of Electrical Engineering and Computer Sciences at University of California at Berkeley in 2006. He spent one year visiting Microsoft Research Redmond as a Postdoc Researcher. He joined the Department of Information Engineering, the Chinese University of Hong Kong, in 2007, where he is now an Associate Professor. He is also currently an Adjunct Associate Professor in Tsinghua University, Institute of Interdisciplinary Information Sciences. He received the Eli Jury award from UC Berkeley in 2007 (presented to a graduate student or recent alumnus for outstanding achievement in the area of Systems, Communications, Control, or Signal Processing) and The Chinese University of Hong Kong Young Researcher Award in 2013. He also received several best paper awards, including IEEE ICME Best Paper Award in 2009, IEEE Transactions on Multimedia Prize Paper Award in 2009, and ACM Multimedia Best Paper Award in 2012. He serves as TPC Co-Chair, General Chair, and Steering Committee Chair of ACM e-Energy in 2016, 2017, and 2018 - present, respectively. He will serve as TPC Co-Chair for ACM Mobihoc 2020. He also serves as Associate Editor of IEEE/ACM Transactions on Networking in 2014 - 2018. He receives the ACM Recognition of Service Award in 2017 for the service contribution to the research community. His recent research interests include energy systems (e.g., smart power grids and energy-efficient data centers), intelligent transportation systems, distributed optimization, data networking, delay-constrained communication, machine learning in networked and societal systems, and capitalizing the benefit of data-driven prediction in algorithm/system design.